ChatGPT “hallucinations”: 5 reasons human expertise is even more critical
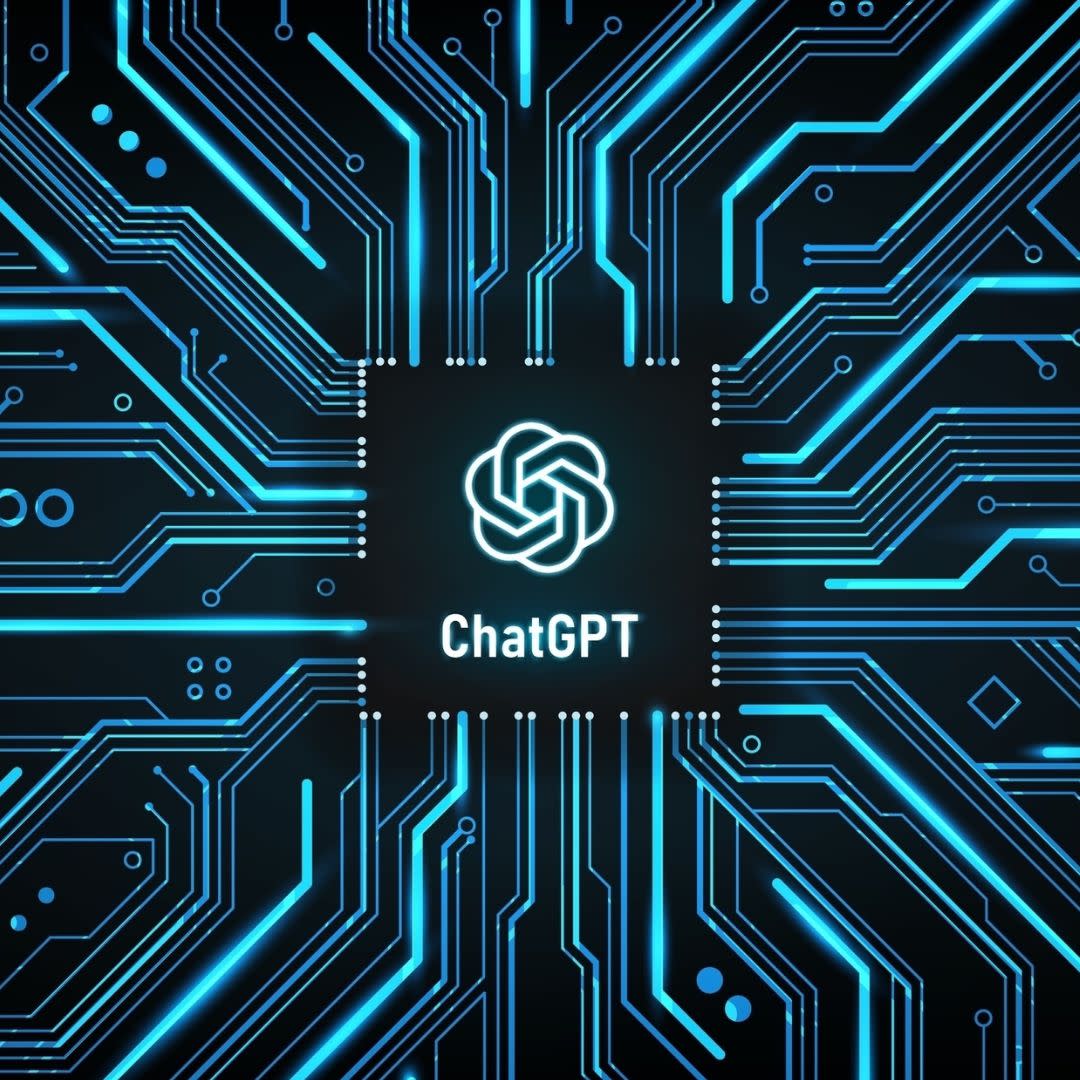
by NotedSource
In today's competitive business environment, corporations constantly look for ways to innovate and stay ahead of the curve. Many professionals in research, writing, or varying business roles are turning to solutions like ChatGPT or Google's Bard to enhance their ability to conduct research at scale and then summarize it. However, more and more frequently, professionals using AI to conduct research and write are experiencing ChatGPT “hallucinations." These hallucinations cite made-up information and have resulted in the loss of professional licenses, jobs, and prestige for those who have submitted work products that include the hallucinations.
Hallucinations in the context of language models like ChatGPT refer to situations where the model generates plausible-sounding responses but are factually incorrect, nonsensical, or unrelated to the input. These hallucinations occur because language models like GPT-3.5 lack true understanding and only create responses based on patterns and associations learned from the training data.
There are several reasons why hallucinations occur in AI language models:
-
Lack of real-world understanding: Language models need to possess true comprehension or
awareness of the world. They merely mimic patterns from the data they were trained on, so they can generate responses that sound sensible but are nonsensical or inaccurate.
-
Bias in the training data: Language models are trained on vast amounts of text data from the internet, which may contain biased or incorrect information. Consequently, the model can produce biased or misleading responses.
-
Over-optimization of training data: Language models are trained to predict the most likely next word given the context in the training data. If a particular response is commonly found in the data, the model may over-attribute likelihood to that response, leading to repeated or overconfident generating of certain outputs.
-
Absence of context: GPT-3.5 has a context window, which means it can only consider a limited amount of text before generating a response. The model may provide irrelevant or nonsensical answers if important context is omitted.
The presence of hallucinations emphasizes the crucial role of human expertise and annotated references in using language models effectively and responsibly.
-
Fact-checking and verification: Human experts play a critical role in fact-checking and validating the responses generated by language models. They can cross-reference the information provided by the model with trusted sources to ensure accuracy.
-
Identifying bias and ethical considerations: Human reviewers can identify and address potential biases in the model's responses. They can also ensure the language model adheres to ethical guidelines and doesn't propagate harmful content.
-
Training data curation: Experts can curate the training data to include diverse and reliable sources, reducing the likelihood of the model hallucinating false information or biased responses.
-
Contextual understanding: Human experts can provide essential context that the language model may lack, leading to more accurate and contextually relevant responses.
-
Improving the model: Feedback from human experts and users can be used to fine-tune and improve the language model, reducing the occurrence of hallucinations and enhancing its overall performance.
While language models like ChatGPT can be powerful tools, they are not infallible and should always be used with human expertise. Ensuring that human expertise is always consulted is critical to ensure the highest quality of outputs and minimize the risk of spreading misinformation.
One way to do this is to contract with academic researchers.
Academic researchers are constantly conducting cutting-edge research that can significantly benefit corporations. They have specialized expertise in various areas, including engineering, science, medicine, and business. By working with academic researchers, corporations can tap into new ideas and perspectives, developing new products, services, and processes.
In addition to the benefits of access to cutting-edge research and specialized expertise, contracting with academic researchers can also help corporations to improve their reputations. By working with academic researchers, corporations can demonstrate their commitment to innovation and willingness to invest in research and development.
Of course, there are also some challenges associated with contracting with academic researchers. For example, finding academic researchers working on suitable topics can be challenging. Additionally, managing the relationship between the corporation and the academic researchers can be difficult. However, the benefits of contracting with academic researchers outweigh the challenges.
Fortunately, NotedSource is a platform that connects businesses with a network of highly qualified academics and professors for short-term R&D projects. The NotedSource platform matches businesses with the right academic experts for their specific needs. The platform also provides several features that make it easy for companies to manage projects, communicate with team members, and track progress.
As innovation accelerates, more corporations will likely turn to AI language models. By engaging academic researchers, businesses can also ensure that they mitigate the risk associated with AI while still using the latest in tech and research to develop new products, services, and processes.